As recently as five years ago, many health marketing firms lacked an in-house data operation. Now nearly every organization has multiple data scientists on call — and, with the arrival of generative artificial intelligence models such as ChatGPT, these operations have become more sophisticated.
But with this increase in sophistication has come yet another shift in traditional data- and analytics-oriented roles. It’s as likely as not that an organization’s top data person has already been tasked with a range of AI-related responsibilities, most notably as prompt engineer.
We asked four data/analytics pros to tell us how the traditional data scientist role is evolving to accommodate these new types of assignments.
Sayee Natarajan, president and CEO of RxDataScience, which Syneos Health acquired in 2021, acknowledges the obvious right up front: that, since the debut of ChatGPT and large language models in general, most companies’ approach to AI has changed. “Everyone is surprised at how well the models work and how fast they’re maturing. We’re just beginning to grasp how this will change every single person’s job,” he says.
Until now, Natarajan notes, people working in data needed to understand some technology, the domain and the data. But today, those same people need to be able to write a well-constructed AI prompt.
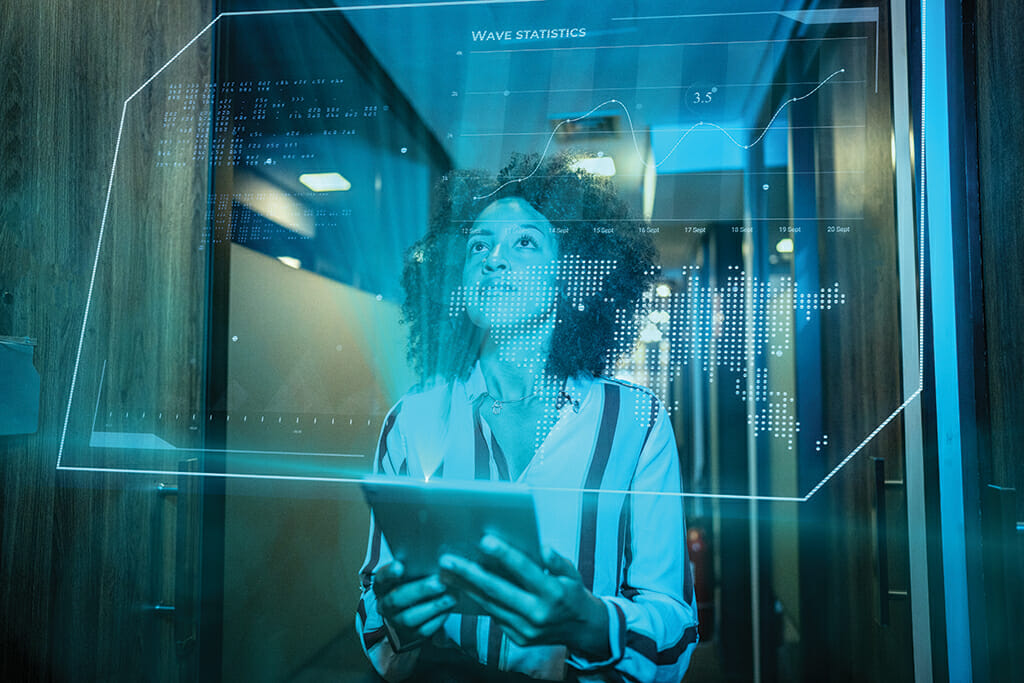
“AI is very sensitive, and if you use the wrong language, it doesn’t answer the question properly,” he explains. “The ability to rephrase a sentence repeatedly is a very useful skill. You cannot succeed if no one on your team can write well.”
That’s why Natarajan has expanded his team to include data aces and technologists who understand processes and workflows. “AI is becoming easier to program. So now the limit is not so much how well you can write code, but more how well you understand the problem and can communicate it — not only to the LLM, but to the stakeholders as well.” (“Large language model” is a general term for AI technologies such as ChatGPT.)
As for fears that generative AI will put people out of work, Natarajan believes they have been overstated. “AI will reduce employee workloads, but that means you have more capacity. Now it’s up to your team to invent something new, to be more productive. Once you’re no longer wasting your brainpower on mundane, mind-numbing stuff, how much more brilliant will you be at your job?”
“This has been the craziest year of my career in terms of how fast developments are coming,” Natarajan continues. “We can’t predict how it will evolve, but humans have always been able to adapt.”
Christina Kim, Omnicom Health Group’s chief strategy and analytics officer, reports that AI has become a part of nearly every client conversation: “It’s an important component of our workflow to help scale efficiency.” At the same time, she cautions that the transition to the AI era isn’t as simple as flicking a switch. Time and resources must be devoted to the comparatively unexciting groundwork.
“Besides providing access to the tools, we’re educating all of our people about what it really means to apply AI and how AI can impact our work,” she notes.
Kim says that data scientists looking to take on a bigger role in AI-related projects must, first and foremost, learn to distill the problem the organization is trying to solve. Flexibility, as always, remains essential.
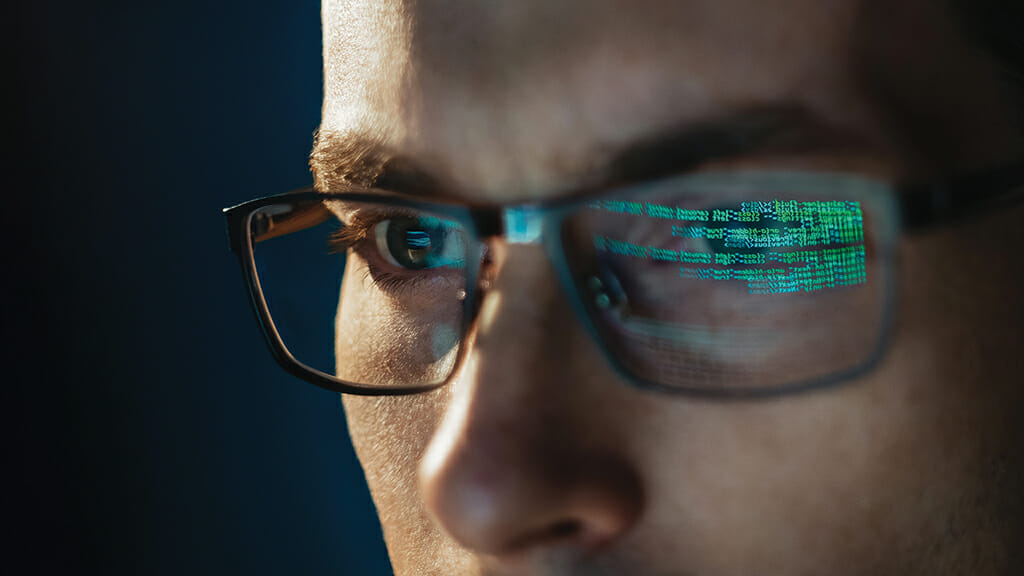
“AI takes a lot of fine-tuning. You have to adjust the model to get the output that addresses the problem,” she explains. That’s why she believes the data scientist role “will become increasingly collaborative. To customize these models, they’ll need to understand the business across all the different teams.”
As for where the most robust opportunities lie, Kim points to personalized medicine: “We’ll be using our behaviors, genetic makeup, medical history, treatment preferences and family backgrounds to develop a personalized treatment plan. Then you can sift through the patient’s and the insurer’s financial responsibility, and figure out how to make that treatment plan cost-effective.”
She also sees AI as “a great equalizer in terms of getting folks trained in healthcare marketing. It will supercharge some jobs and create more opportunities for other roles that are leveraging AI.”
Still, Kim doesn’t anticipate too many data scientist jobs going the way of the dodo bird. “You’ll never really take away the code responsibility from data scientists,” she adds. “But their focus will be more on deriving insights from AI models and applying those insights to the business.”
Seth Reeser, Calcium’s VP, associate director, software and dev-ops, points out that the platforms used for data analytics now commonly include prebuilt AI or machine learning models. This, he believes, opens the door to a wider range of users: “What once had to be created manually or in-house is now being developed for you, and pretty much any traditional marketer can use them.”
But Reeser cautions that the health industry — not to mention its marketing apparatus — is still very much in its infancy in terms of its understanding of machine learning.
“There’s still a lot to explore in healthcare,” he explains. “We’re finding ways to incorporate AI into products and services, and also educating clients about it. And there are nuances in healthcare, of course, such as creating models using data that may include PHI [protected health information]. A lot of times we anonymize data so they’re more of an aggregate.”
Traditionally, the data scientist role demanded a strong statistical background, but Reeser believes this is no longer a prerequisite. “It could be as simple as training someone in a marketing platform that already has built-in AI and machine-learning capabilities,” he continues. “We’re transitioning a lot of traditional data analysts and data strategists into having the skill sets of a data scientist in our industry.”

In other words, more change is imminent. “The big push now is to incorporate AI into everything we do, so I expect the complexities and skill sets to shift,” he adds. “I might reminisce about the old days or the simple life, but I will say that we’re propelling the industry and the healthcare therapeutic options, which is everyone’s primary mission. It’s a new era.”
Fred Bennett, BGB Group’s chief digital officer, notes that while the tools used by data scientists are evolving, the overarching mindset remains very similar. “The enduring things that make a scientist — the approach, the rigor — are still critically important to us as we evaluate talent.”
Of course, familiarity with the already accumulated data doesn’t hurt. “You have to be able to understand what the data is and what it isn’t,” he explains. “There are risks to having sophisticated models take the data and run with it.”
Bennett doesn’t view AI as a potential panacea. In fact, he urges restraint. “A lot of these analyses mask the underlying data, making it hard to trace back and see the causality. There’s also the risk that people will take the output as gospel without knowing all the caveats,” he adds.
Essentially, all results need to withstand critical analysis — and the more complex the tools used to generate them, the harder it can be to verify the output.
Like Natarajan, Bennett doesn’t believe the increasing prominence of AI will result in too many data scientists kicked to the curb. “For any job that’s replaced, others are created,” he says. “Today, you can take college classes in prompt engineering, which is the art of optimizing the prompts you’re giving your AI tools. Just six months ago, that wasn’t even a thing.”
In fact, he thinks companies might need even more data scientists under their roofs. “At the largest companies, there’s an opportunity for specialization. People can go deep into specific areas of expertise,” Bennett notes. “For a small biotech where one person in the analytics department is wearing multiple hats, it makes sense to have someone dedicated to AI. Those tools require a specific skill set.”
Still, he stresses, there are likely to be bumps on the road ahead. “In some ways the technology is well understood, but we’re still in the steep part of the learning curve regarding its applications,” he adds. “As creative people and marketers and pharma company people, we need to be asking, ‘How can we do things better using these new tools?’ This is an area that will definitely keep evolving.”
From the September 01, 2023 Issue of MM+M - Medical Marketing and Media