All the recent “AI is coming to healthcare marketing” chatter tends to overlook one important piece of information: It’s already here. Organizations big and small across a range of therapeutic categories are using tactics grouped under the AI umbrella — machine learning, natural language processing and the like — to great effect. Anyone who says otherwise is either out of the techno-loop or attempting to preserve some imagined competitive advantage.
Where there seems to be a disconnect is in expectations around the use of these tactics. Perhaps because of the hype, there’s a sense that AI will magically transform an organization’s mess of data into beautifully ordered stacks that are ripe to be mined, which couldn’t be further from the case. AI tactics are only as useful and potentially effective as the data that fuels them.
Similarly, it goes without saying that certain organizations are more forward-minded than others, both in their ability to collect/parse zettabytes of data and to extract actionable insights from it. And even within the companies that are “good at data,” there are often splits: An ability to tap into this information at an organizational level doesn’t necessarily correlate to an ability to tap into it at a brand level.
Finally, there’s the question of resources. If they’re so inclined, big-name pharma organizations can afford to import an army of data scientists or partner with the A-list of analytically attuned agencies. There are exceptions — see the first case study below — but upstart biotechs with only one or two molecules generally lack the money and energy to infuse their marketing operations with similar expertise.
“The level of industry maturity in a big-picture sense? It’s spotty but improving,” says Hans Kaspersetz, CTO of Cherry Healthcare Communications and president, chief strategist of Arteric, which has worked on AI-intensive programs for AstraZeneca and Apellis Pharmaceuticals, among others. “The [companies] that do it well tend to do it really well.”
MM&M talked with organizations willing to share details of recent AI-infused programs, especially ones that tapped AI tactics to solve specific and narrowly defined problems. Here are three that stand out for their ingenuity.
Apellis, Cherry Healthcare Communications and Arteric: Solving a search-related dilemma
When Apellis first engaged Kaspersetz’s team, it was for a relatively traditional assignment: helping identify and locate ideal candidates for clinical trials in difficult-to-reach patient populations. After the team delivered on its promise, the companies expanded their relationship to include a data-intensive examination of Apellis’ content around a specific condition — a kind of new-age competitive analysis.
The companies set about conducting what Kaspersetz calls a “word-embedding” project designed to gauge Apellis’ authority across a range of topics compared to its competitors. To kick-start the engagement, the team used machine learning to analyze data and information about multiple disease states published on the corporate websites of Apellis and the designated competitors.
After analyzing the data, Kaspersetz and his team identified gaps in Apellis’ content across those disease states on its corporate site. While Apellis’ investor relations web hub was packed with information about them, it didn’t accrue the same equity in search as the corporate site. Essentially, this meant Apellis — the rare biotech with the will and resources to conduct such self-analysis — wasn’t taking full advantage of opportunities to position itself as an authority in and around those disease states.
“They were sure they should rank higher. Sure enough, there was a reason they didn’t,” Kaspersetz explains.
While in this instance the client was thrilled to have answered a specific question using AI tactics, the results often are not received quite as warmly. “This kind of work and analysis has a tendency to uncover all the warts, because it’s not subjective in nature,” Kaspersetz says. “You’re not asking somebody, ‘How do you feel about this content? Do you think the content is well-written?’ Sometimes looking at things in a purely mathematical way is divorced from ‘this is a good campaign.’”
Pfizer Oncology and ghg: Helping oncologists parse an essential volume
Pfizer Oncology’s Global Status of Advanced/Metastatic Breast Cancer 2005-2015 Decade Report compiled a treasure trove of information about treatment of the disease, identifying care advances as well as new avenues for access and support. It was one of the most comprehensive volumes on the disease produced in recent years.
It also ran hundreds of pages long, with dense copy that didn’t exactly lend itself to beach reading. This posed a problem: Even those physicians with a keen desire to peruse the volume only have so many hours in a day.
“Getting that kind of information into people’s hands is always very challenging, but it’s especially challenging in the HCP space,” says Chris Millsom, SVP, digital strategy director at Wunderman Thompson Health, who held the same post at ghg prior to the agency’s merger into WTH. “We needed to make it faster and easier for [oncologists] to access it.”
To facilitate consumption of the report, Pfizer and ghg partnered with IBM Watson. Together, they built mBC|With Watson, which employed natural language processing to allow physicians to, essentially, ask the report questions using common conversational language.
It no longer takes months and millions of dollars to build things like this. They’re easier conversations to have.
Chris Millsom, Wunderman Thompson Health
Pfizer chose to debut mBC|With Watson at a high-profile oncology event: the San Antonio Breast Cancer Symposium. While the ghg team had some trepidation about managing expectations — “we were worried that people would expect it to be able to answer pretty much any question about anything,” Millsom recalls — it was received exceedingly well by the conference throng.
“It made [accessing information in the report] so much easier,” he continues. “It opened a lot of eyes.”
The success of the mBC|With Watson program had a cascade effect, opening the door for ghg (and Wunderman) to propose AI and tech-enabled solutions to other, more hesitant clients. “It no longer takes months and millions of dollars to build things like this,” Millsom says. “They’re easier conversations to have.”
Sage Therapeutics and Concentric Health Experience: Diagnosing postpartum depression outside the doctor’s office
For a number of reasons, postpartum depression is difficult to diagnose in the traditional HCP setting. Many of the physicians treating women who recently delivered a child aren’t specifically trained to spot mood disorders. At the same time, those women commonly fail to discuss mood-related symptoms with their physicians.
Responsibility for this lapse occasionally falls on the treating doctors. Some neglect to ask about the woman’s mental and emotional well-being. Other times, they do so in a casual, vague manner (“how are you feeling?”) or fail to avail themselves of mood-disorder questionnaires specifically designed with post-delivery women in mind.
In other cases, it’s the patient herself who paints an incomplete picture of her current emotional state. This is often attributable to the patient’s fear or shame about her symptoms.
Women will talk about physical distress related to exhaustion, hunger, insomnia and the like, but they are much less likely to speak about the purely psychological dimensions of those symptoms.
Brian Dunn, Concentric Health Experience
All of this is why Sage Therapeutics, which was preparing to launch Zulresso, the first drug designed to treat postpartum depression, and Concentric Health Experience set out to better and more quickly diagnose postpartum depression outside the HCP setting. To do this, they turned to natural language processing (NLP), a tactic that has proven increasingly useful to organizations hoping to unearth latent insights within huge volumes of verbiage.
The Sage and Concentric NLP model was trained to sift through billions of public social media posts and flag potential signs of postpartum depression. According to Concentric chief behavioral officer Brian Dunn, data scientists and psychologists trained the algorithm to spot certain words and phrases that could indicate a mood disorder.
Initial revelations included a tendency for women to post about the physical aspects of a mood disorder. “Women will talk about physical distress related to exhaustion, hunger, insomnia and the like, but they are much less likely to speak about the purely psychological dimensions of those symptoms,” Dunn explains. “You don’t see the same disclosure of psychological distress related to intrusive thoughts or pure depressed mood.”
Of course, many new mothers experience exhaustion, fatigue and similar physical symptoms by virtue of, well, their status as a new mother. To account for this, Sage and Concentric trained the NLP model to locate an even more specific identifier of women suffering from postpartum depression: The algorithm looked for superlatives attached to mentions of sleeplessness or exhaustion. Mothers with postpartum depression tended to use words like “worst” or “extremely” in connection with their physical symptoms.
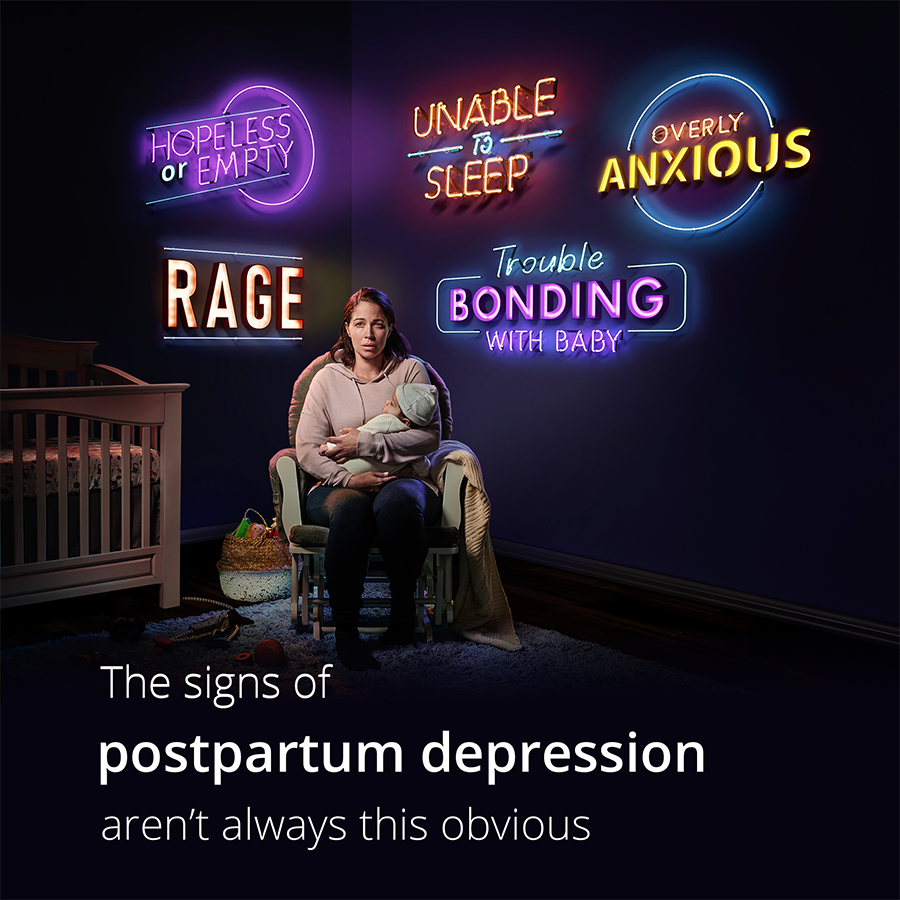
After the algorithm identified signs of depression in social posts, the team used its learnings to inform an awareness campaign, Know the Signs. The landing page for the patient campaign shows a woman and baby surrounded by neon signs with phrases such as “unable to sleep,” “hopeless or empty” and “overly anxious.”
The goal was to show new mothers how postpartum depression might look or sound to them. In tandem with neon lights and a flickering effect, the phrases are meant to stir certain emotions in people who visit the page — a tactic, Dunn says, which is rooted in neuroscience.
“You have a disclosure problem, an ambiguity of symptoms problem and a lack of health literacy problem,” he explains. “People who haven’t had a mood disorder don’t know what to look for. That’s why we get into these subtle analyses that involve teams of data scientists, psychologists, neuroscientists, really good copywriters and creatives.”
AI has the answers
Good artificial intelligence can still, in some precincts, be considered a trade secret. Here are examples of AI success from companies that requested anonymity.
Making sense of semantics:
Back in 2017, one pharma company couldn’t figure out why its in-depth formulary pages were generating almost no web traffic. As it turns out, the company was an unwitting victim of its own precise use of language: Google didn’t view “formulary coverage” and “insurance coverage” as synonyms. “Brands love to talk about formulary coverage because that’s the technically correct way to reference it, but nobody besides brand people and payers call it ‘formulary coverage,’” says the exec charged with remedying the situation. Ultimately,
the agency and client team used natural language processing to bridge all “health insurance” web traffic to the appropriate formulary pages. “It was one of those situations where the solution was blindingly obvious once you identified the problem.”
Directing traffic:
Another pharma company — “a big one, the kind that has the ability to move the market when they make news,” says an exec — wondered why its most recent newsmaking events were followed by an immediate drop in web traffic and search-engine rankings. “Not only weren’t they getting a meaningful bump in traffic, but it was all going down. You’d think, ‘Big event, they win the news cycle’ — nope.”
The exec’s agency used machine learning to micro-analyze the traffic patterns for the week following the news event and learned, much to its amusement, that the client and/or its brands were being crowded out by coverage published by the media organizations favored by Google’s search algorithm — including MM&M. “The company never thought to be a participant in the dialogue around their news, which is ironic on many levels,” the exec says.
— with additional reporting by Alison Kanski
From the September 01, 2019 Issue of MM+M - Medical Marketing and Media